Sep 17
2024
AI Advances Bring RCM To an Inflection Point
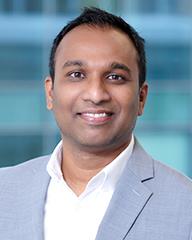
Various forms of automation have long been present within healthcare revenue cycle management (RCM). However, advances in artificial intelligence (AI) have brought the industry to a significant inflection point, where the use cases for AI tools are expanding as rapidly as their capabilities.
We sat down with Thomas Thatapudi, chief information officer of AGS Health, to discuss the current and future state of AI in RCM and what healthcare organizations need to know about effectively integrating it into workflows.
EHR: How are automation and AI reshaping healthcare’s approach to revenue cycle management?
Thatapudi: Healthcare finance leaders have long recognized the power of simple automation, like simple rules-based workflows or analytics dashboards, to improve billing processes and error rates. Now, advanced AI tools like ChatGPT, large language models, and generative AI – or GenAI – have brought RCM to an inflection point with a variety of viable new AI-driven RCM use cases that could have significant financial impacts. AI and automation can reduce manual labor costs and increase net revenue through a seamless process that follows the entire patient journey, from preventing authorization denials upfront and reducing coding errors to implementing more proactive and efficient accounts receivable follow-up processes.
With front-end revenue cycle tasks such as insurance verification and prior authorization, we have an opportunity to create a completely seamless and interactive process for patients while ensuring the presence of appropriate controls to mitigate revenue leakage. For mid-cycle coding, certain specialties lend themselves to autonomous coding that eliminates the need for human intervention, freeing staff to focus on more complex work. On the back end, the focus can shift to denial management and collection rates, particularly for claims that, due to capacity constraints, were left unworked in the past. This can be particularly beneficial in cases where payer requirements have become more stringent.
These examples are just the tip of the iceberg in terms of potential RCM use cases over the next two years.
EHR: What are some examples of areas where AI tools are being used to improve RCM?
Thatapudi: AI is being used in clinical documentation, patient communication and payments, scheduling, prior authorization, and medical coding. In fact, coding has been utilizing true AI and machine learning in the form of NLP-based computer-assisted coding (CAC) for about a decade. With existing CAC applications reaching a plateau in coding accuracies of approximately 70-75 percent, new autonomous solutions are entering the market that leverage deep learning models and Gen AI to truly increase fully automated coding rates. I expect that coding will be one of the RCM areas that will be most heavily impacted by true AI, machine learning, and deep learning.
EHR: How can finance leaders make use of advanced data analytics and business intelligence (BI) tools to inform RCM decisions and measure their impact?
Thatapudi: BI tools can measure a wide range of metrics, from the number of system users to interactions and accounts, all of which can inform the key performance indicators (KPIs) that are crucial for monitoring financial performance. The problem is that the sheer volume of metrics can easily be overwhelming, which can lead to analysis paralysis. To prevent this, it’s important to take a step back and home in on KPIs such as financial indicators like collection rates per day or per month and the time it takes to collect payments—performance indicators that tell how much in time and money is being spent to recover a dollar so it can be reduced or better managed.
It’s important that senior leadership avoid being overly impressed by the wealth of intelligence that can be collected and displayed on a dashboard. The focus should instead be on determining the KPIs that drive day-to-day operational decisions. For example, predictive analytics services help healthcare organizations better predict denials, anticipate underpayments, forecast payments, and more. This allows for proactive claim correction prior to submission, which improves clean claim rates and cash flow. Creating simulations and projections for customized “what-if” scenarios provide an understanding of the impacts associated with interdependent metrics.