Nov 14
2022
RCM and Coding May Help Close Health Equity Gap
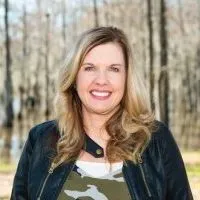
By Leigh Poland, RHIA, CCS, AGS Health.
Health equity is a focus of providers, regulatory agencies, and payers as they seek ways to eliminate care disparities across race and ethnicity, gender, sexual orientation, and socioeconomic status lines. Its significance is further impacted by new quality-based care models beyond those established by the Patient Protection and Affordable Care Act of 2010.
The challenge for many healthcare organizations participating in these new reimbursement models is how to view health equity and social determinants of health (SDoH) to understand the actual value of this information. Often overlooked is that healthcare organizations’ coding and revenue cycle management (RCM) departments already aggregate information that can help better understand inequities in care delivery and health equity across their patient populations.
A Primer on SDOH Impacts
SDoH impact many health risks and outcomes, which is why this data is vital for clinical care and reimbursements. Defining factors can include anything from geography, race, gender, and age to disability, health plan, or any other shared characteristic. Of increased importance, SDoH issues are most often experienced by the most vulnerable members of society: the poor, less educated, and other disadvantaged groups.
SDoH is linked negatively with outcomes, including higher hospital readmissions, length of stay (LOS), and increased need for post-acute care. Value-based payment programs, therefore, may penalize organizations that disproportionately serve disadvantaged populations if they do not collect and respond to SDoH data.
For example, addressing food insecurity — a key SDoH data point — by connecting patients to programs like Meals on Wheels, Supplemental Nutrition Assistance Programs (SNAP), or food pantries is proven to reduce malnutrition rates and improve short and long-term health outcomes.
In the case of SNAP, which is the primary source of nutrition assistance for more than 42 million low-income Americans, participants are more likely to report excellent or very good health than low-income non-participants. Low-income adults participating in SNAP incur about 25% less medical care costs (~$1,400) per year than low-income non-participants.