Jan 29
2022
Artificial Intelligence Tools Have Immense Potential To Improve Overall Patient Care
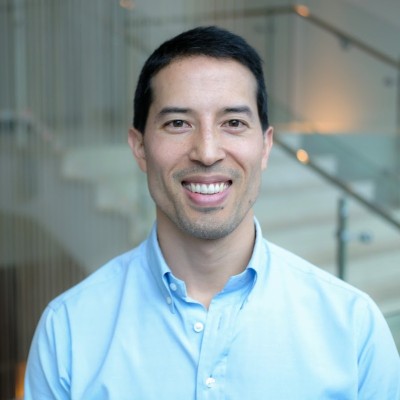
By Andrew Pucher, CEO, Dascena, Inc.
Artificial intelligence (AI) is poised to have a transformative impact on the delivery of healthcare. The COVID-19 pandemic has brought to light seemingly insurmountable challenges in the healthcare system including, shortages in medical supplies, scarcity of hospital beds, and overflowing ICUs and emergency departments. Combined, these challenges have caused increased stress and burnout in healthcare providers (HCPs).
Although the issue of nursing shortages and burnout were well documented in the U.S. before the start of the pandemic, the unprecedented influx of patients within a short time span has exacerbated this issue, resulting in a mass exodus of nearly half a million healthcare workers since February 2020. The severe shortage of healthcare workers has led to an immense strain on the entire healthcare industry, ultimately impacting patients in need of both urgent and essential medical care.
Although still in a nascent stage, AI-based systems hold much promise for alleviating some of these burdens by improving individualized healthcare delivery to patients. This technology is gradually being adopted by healthcare providers to assist and supplement the standard clinical workflow. However, with the current state of the pandemic, it is imperative to consider appropriate measures to accelerate the implementation of these systems to improve patient outcomes.
Setting the Stage for AI and ML in Healthcare
The past decade has seen significant improvements in computational power, the development of powerful machine learning techniques, and a growing abundance of ‘big data’. Scientists have harnessed the power of these resources to develop innovative tools such as continuous monitoring devices and genetic screening tools which enable personalized healthcare. These advances have collectively propelled research in the field of AI in medicine to new heights.
Machine learning (ML), a branch of AI, focuses on the development of systems that are capable of learning from data. The development of ML-based clinical decision support (CDS) tools involves the initial training of a ML algorithm (MLA) with large electronic health record (EHR) datasets to analyze and “learn: complex trends from a vast number of input variables – like patient data and demographics – with the goal of identifying or predicting the onset of a specific medical condition or outcome. Following testing and validation, these systems are able to distinguish trends in the data and make assessments and recommendations without human intervention.