Jan 29
2022
Artificial Intelligence Tools Have Immense Potential To Improve Overall Patient Care
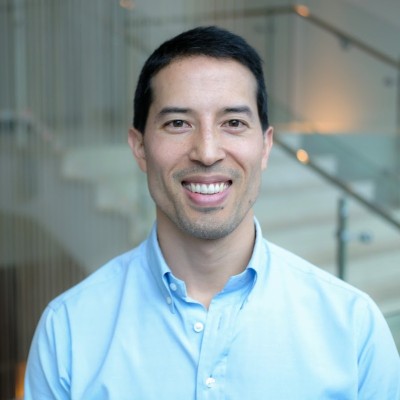
By Andrew Pucher, CEO, Dascena, Inc.
Artificial intelligence (AI) is poised to have a transformative impact on the delivery of healthcare. The COVID-19 pandemic has brought to light seemingly insurmountable challenges in the healthcare system including, shortages in medical supplies, scarcity of hospital beds, and overflowing ICUs and emergency departments. Combined, these challenges have caused increased stress and burnout in healthcare providers (HCPs).
Although the issue of nursing shortages and burnout were well documented in the U.S. before the start of the pandemic, the unprecedented influx of patients within a short time span has exacerbated this issue, resulting in a mass exodus of nearly half a million healthcare workers since February 2020. The severe shortage of healthcare workers has led to an immense strain on the entire healthcare industry, ultimately impacting patients in need of both urgent and essential medical care.
Although still in a nascent stage, AI-based systems hold much promise for alleviating some of these burdens by improving individualized healthcare delivery to patients. This technology is gradually being adopted by healthcare providers to assist and supplement the standard clinical workflow. However, with the current state of the pandemic, it is imperative to consider appropriate measures to accelerate the implementation of these systems to improve patient outcomes.
Setting the Stage for AI and ML in Healthcare
The past decade has seen significant improvements in computational power, the development of powerful machine learning techniques, and a growing abundance of ‘big data’. Scientists have harnessed the power of these resources to develop innovative tools such as continuous monitoring devices and genetic screening tools which enable personalized healthcare. These advances have collectively propelled research in the field of AI in medicine to new heights.
Machine learning (ML), a branch of AI, focuses on the development of systems that are capable of learning from data. The development of ML-based clinical decision support (CDS) tools involves the initial training of a ML algorithm (MLA) with large electronic health record (EHR) datasets to analyze and “learn: complex trends from a vast number of input variables – like patient data and demographics – with the goal of identifying or predicting the onset of a specific medical condition or outcome. Following testing and validation, these systems are able to distinguish trends in the data and make assessments and recommendations without human intervention.
Benefiting from machine learning in resource-constrained environments
ML-based CDS tools have immense potential to improve overall patient care in several ways. First, traditional clinical scoring systems and risk stratification tools – ones that have been used by clinicians for decades – rely on periodic monitoring and labor-intensive manual entry of clinical features of hospitalized patients. In contrast, ML-based CDS tools can be seamlessly integrated into existing hospital EHR systems and run passively in the background.
They are capable of continuously analyzing routinely collected patient data without requiring any additional supervision or inputs from clinical care teams. These tools can perform complex analyses of dynamic trends and interactions of multiple input features including vital signs, clinical measurements, and lab results from individual patients. Based on this analysis, they can serve as a screening tool to detect the onset of life-threatening medical conditions, such as sepsis.
These autonomous tools can greatly benefit clinicians in any environment. However, the benefits are amplified in clinical settings that are strained for resources as a result of the ongoing pandemic, and where continuous monitoring of hospitalized patients is limited by the shortage of nurses on duty. Once high-risk patients for a particular condition have been identified by the CDS system, clinicians are provided with a notification.
These notifications can be tailored to meet the needs of clinical care teams and hospitals to maximize their potential value and reduce alert fatigue. For example, some health systems may prefer to receive their alerts directly within the EHR, while others may prefer faxes or text messages.
These alerts allow care teams to focus on patients that require urgent medical attention. In the context of the current global health emergency, where nurses have limited bandwidth and are overburdened by the sheer volume of hospitalized patients, such a CDS system may improve resource allocation and aid clinical decision-making, which could ultimately lead to improved patient outcomes.
Another key advantage of utilizing ML-based CDS tools is that they can be designed to predict the onset of a medical condition hours before the condition can be clinically detected. Unlike humans, CDS tools can identify subtle variations in patient features associated with the future onset of a specific medical condition and provide alerts to clinicians as to the patient’s disease trajectory.
This provides an opportunity for clinicians to take early action and implement appropriate prophylactic measures to preclude the deterioration of the patient’s condition. As complications arise and a patient’s condition declines, the amount of both material and personnel resources allocated to the patient increases. Thus, ML-based CDS tools would enable more efficient management of resources and allow healthcare providers to focus scarce resources on critically ill patients.
In addition to CDS tools, AI systems are increasingly being adopted for several other procedures such as appointment scheduling, diagnostic imaging, and patient management. Collectively, these tools can aid in streamlining daily operations, enhance information sharing between clinical care teams and improve overall decision-making. Such improvements could increase productivity whilst simultaneously decreasing the burden on medical practitioners, leading to improved patient outcomes.
The bottom line
The pandemic has highlighted significant gaps in not only the U.S., but the global healthcare infrastructure. This is a critical juncture for the healthcare sector and the industry as a whole to embrace and acknowledge the potential of AI to alleviate some of the burdens being experienced by HCPs.
However, there are still many regulatory obstacles and justifiable concerns regarding the widespread deployment of ML-based CDS tools, including data privacy, generalizability of models, and biases in the models. As we look toward the future, identifying legal and ethical frameworks for implementing robust, flexible and secure tools will be critical for transforming workflows as well as patients’ lives and outcomes.