Aug 5
2013
Crowdsourcing Medical Decisions: Putting Big Data to Work in Healthcare
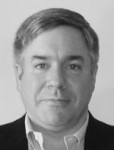
Health Information Technology for Economic and Clinical Health Act
Guest post by Richard Cramer is Informatica‘s Chief Healthcare Strategist.
The widespread adoption of electronic health records has been a key objective of the Health Information Technology for Economic and Clinical Health (HITECH) Act, enacted as part of the American Recovery and Reinvestment Act of 2009. With the pervasive use of these electronic health records, an enormous volume of clinical data is now becoming readily accessible that has previously been locked away in paper charts.
The potential value of this data to yield insights into what works in healthcare, and what doesn’t work, dwarfs the benefits of simply replacing a paper chart with an electronic system. There’s appropriate enthusiasm that this data is going to be a veritable goldmine for enterprise data warehousing, business intelligence, and comparative effectiveness research. However, there are other, equally valuable, uses for this data to enhance clinical decision-making and improve the value of healthcare spending. Simply having instant access to large volumes of data that span thousands or tens-of-thousands of physicians, hundreds-of-thousands of patients and millions of encounters, offers an unparalleled opportunity to increase the quality and lower the cost of healthcare.
Some of you may remember a memorable video from a TED conference where the audience is asked to estimate the weight of a live ox on stage. Although the guesses from the audience varied from unrealistically low to strangely high, the average actually comes within three pounds of the correct weight. The point here is that the collective intelligence of a crowd can answer a question with greater accuracy than an expert in ‘ox weight estimating’ could achieve individually.
When taking this concept to an electronic medium – namely internet searches – and greatly expand the number of opinions, great things can be done with the data that would otherwise be impossible to achieve through normal intellectual processes.
For example, most everyone is familiar with the Google functionality that offers alternative “Did you mean…” suggestions to a search term that has been entered. What may not be so obvious is there is no explicit definition of the alternative search phrase suggestions behind this functionality. Rather, simple “crowdsourcing” of end-user searches is what comes into play.
While technically complex to implement at scale, the process is logically quite simple. Google knows what search phrase was entered, and Google can infer if the search results were what the user intended by evaluating the user’s actions after the search results are displayed. If the user clicks on one of the returned links, then Google knows the search phrase was at least minimally valid.
Alternately, if the user sees the results of a search, but then goes back and changes the search phrase – either to correct misspellings, make the search more broad or more specific – Google knows that the initial search phrase was likely off target. In more complex fashion, Google can also derive the same insight if the user modifies the search phrase even after following some of the returned links and not finding the intended information.
So whenever the search phrase is modified it becomes a candidate for a “Did you mean…” suggestion. Do this a few hundred million times and you can crowdsource some pretty darn accurate suggestions that tolerate all types of data entry errors (spelling, word sequence, word omission, specificity, etc.) as well as logic errors (using the wrong search phrase for the desired results). Google accelerates getting to the best search phrase by offering suggestions that bypass intermediate steps. And the most powerful part is that the suggestions are algorithmically derived from available data rather than having to be explicitly defined through consensus building and programming.
Let’s now circle back to earlier starting premise about the data that will be available as a result of the widespread adoption of EHRs. It’s relatively straightforward to envision a day when a doctor or other care provider is entering information about a patient into an EHR, and they see an alert that says “Have you considered…”
These suggestions could include questions to ask the patient to gather additional information, diagnostic tests or studies to aide in diagnosis, or interventions that might yield higher value results. This will have been driven in the background by crowdsourcing the decisions of thousands of other providers who have seen patients with similar characteristics. This type of alerting can be a natural consequence of documenting electronically a typical patient visit – knowing who the patient is (age, gender, ethnicity, etc.), subjective findings (pain, rash, swelling), objective findings (lab results, other studies) etc.
For patients who are seeing their provider with a similar complaint, what tests did other doctors order to help in the diagnosis? Given similar patients with specific subjective and objective findings, what diagnoses were made? What interventions were prescribed (drugs? surgery?).
And ultimately, what course of treatment resulted in the best outcomes? And unlike the ox weight example from the TED conference, or Google search suggestions, the analysis is being based upon the prior decisions of other healthcare experts – not the general population.
Crowdsourcing the collective intellect of a large group of providers based on vast numbers of patient encounters directly addressed this issue. Far from telling the provider what to do, this type of approach simply harnesses the collective intelligence of the entire medical community to offer alternatives that might not have otherwise been considered. Alerts can be delivered interactively, at the point-of-care, where they can make the most impact in influencing a clinician’s decision-making. And the computer isn’t telling the doctor what to do. In essence, care providers are being provided with a crowdsourced “virtual consult” on every patient, at every step of the care process, to help deliver higher value care.
Today, best clinical practices are most often derived through randomized clinical trials or expert opinion – yet neither approach addresses even a small fraction of the scope and complexity of patient and clinical variation that occurs in the real world.
To be clear, I am not suggesting crowdsourcing EHR data as a replacement for traditional data warehousing and analytics approaches that form the basis of comparative effectiveness research. Rather, by applying data processing algorithms and techniques that are well proven in commercial applications, we have the opportunity to quickly implement solutions with the potential to dramatically change the cost/value/efficacy curves in healthcare the same way similar approaches have been proven to work in other industries.
Oh, and one more thing. Sometimes Google doesn’t say “Did you mean…”
Instead it just does the search the end user should have done, simply because it knows better.
Richard Cramer is Informatica’s Chief Healthcare Strategist. He is a passionate advocate for the role that information technology and data can play in improving the quality and value of healthcare in the United States. Working closely with senior clinical and business leaders – representing payers, providers and technology companies – Richard maintains a comprehensive and poignant point of view on how organizations can move from current state systems and processes, to a healthcare future where care is high quality, high value and data-driven. Immediately prior to joining Informatica, Richard received a Bachelor of Science degree in computer science from the United States Air Force Academy and prior to his time in healthcare served as a flight test engineering and systems analyst.
One comment on “Crowdsourcing Medical Decisions: Putting Big Data to Work in Healthcare”