Nov 14
2019
Taking Predictive Analytics In Healthcare From The Experimental To The Expected
By Marc Helberg, managing vice president at the Philadelphia office, Pariveda Solutions.
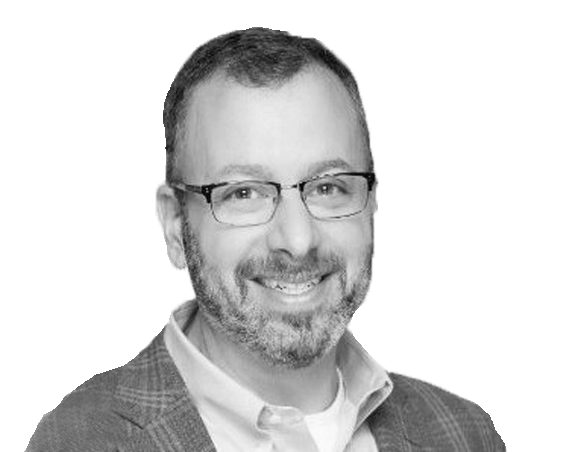
Data. It’s everywhere, and healthcare organizations know it can be theoretically useful in the modeling of predictive patient outcomes.
This sounds promising enough. But when we look beneath the surface, we learn that the industry as a whole has been relatively slow to adopt predictive analytics as a standard course of nonacademic care. In fact, just 34% of providers include it as part of their strategy.
This isn’t to say there aren’t plans for future applications. Providers recognize the potential for predictive analytics to improve outcomes and lower long-term costs. A Deloitte survey found that 84% of executives certainly see the benefits of predictive analytics in healthcare, as they say it will play a role in their organization’s strategy over the next three years.
So when will the role of data analytics in healthcare move from academic to more practical applications? And perhaps more importantly, what stops providers from taking action?
The Promise of Predictive Analytics
Some of the hesitation about analytics can be credited to misunderstandings about its scope. Given the hype — the market is expected to nearly quadruple in size to $8.46 billion by 2025, according to some estimates — many providers believe predictive analytics will make inherently disruptive changes to day-to-day operations. This includes supplanting sensitive tasks usually carried out by doctors (such as developing custom treatment plans).
In reality, analytics should focus on incremental improvements to allow doctors to do more of what they already do well. As it stands, six out of 10 physicians feel their time with patients is far too short to treat them effectively. The median time for visits is 11.8 minutes for patients in their 30s and rises to 14.3 minutes for patients who are 70 or older.
With predictive analytics, one possible initial deployment could assist with calendar management by using data on missed or canceled appointments to help doctors better estimate when they might have more time to handle complex patient visits. Small changes like this one could make a significant impact on patient experience.
Even if a provider is willing to invest in a larger-scope analytics project, its value will come in augmenting existing processes, not redoing them. In the near future, patient-generated data could help doctors assess and score the risk of an illness or disease in particular cases, but it will still require a doctor’s expertise to interpret the best way for the patient to address that risk.
When applied during care delivery, analytics could even factor in social and environmental conditions that could increase the likelihood of complications. That alone could reduce hospital re-admissions and improve the standard of care across the spectrum.
Again, this has nothing to do with a patient’s treatment plan. There will always be a need for human creativity in care that only a doctor or clinician can provide. Instead, the pros and cons of predictive analytics in healthcare can be better divided into high- and low-value applications. Data can excel in identifying health challenges, anticipating certain risk factors, and streamlining administrative tasks, which also provides an opportunity to further improve patient care.
Although barriers still prevent fully implementing predictive analytics in healthcare, leaders — especially those in information technology — can help break them down. Here are some of the best ways to start:
- Organize your data. Providers have long struggled to manage the data necessary to implement predictive analytics. One survey suggests that alongside budget and regulatory challenges, data management is one of the most consistent barriers to implementing more analytics.
To put this in perspective, let’s consider diabetes. Dozens of data markers go into its prevention: sugar intake, diet, exercise, etc. But until that data is connected, organized, and understandable to a program powered by artificial intelligence, you can’t truly explore the value analytics play in preventing it.
- Increase monitoring. Sometimes, the simplest of strategies can bring about the biggest results. Monitoring falls squarely within this camp. To the first point, it’s the best way to collect useful data.
If your patient has a device to track blood pressure, for example, you could deploy an analytics program to identify any relationship between blood pressure spikes and specific daily events (such as travel or vigorous exercise). Armed with this information, doctors could provide more tailored advice for patients.
- Encourage open communication between the tech department and doctors. Despite the potential benefits of implementing predictive analytics in healthcare, many doctors who could benefit from it aren’t ready to take the leap due to concerns about patient safety.
Many providers have yet to figure out how to reassure staff that emerging technology won’t harm patients or take over their work, and IT teams are best suited to lead those conversations. They should communicate in a language doctors and their staff can understand. Educating them about how predictive analytics can expand the scope of care and bring a broader base of knowledge to better treat individuals is a great way to start.
Bringing predictive analytics into practical rather than theoretical applications is no small feat, and there are many stakeholders that must buy in. However, achieving this isn’t impossible. You have the facts on your side — it’s time to start communicating them.