Aug 10
2020
Diagnosing Bias In Healthcare AI: Five Best Practices
By Carlos Meléndez, COO, Wovenware.
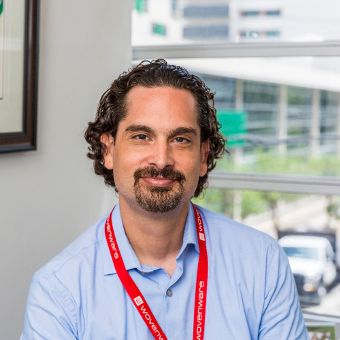
A recent Wall Street Journal article pointed to a biased algorithm widely used in hospitals that unfairly prioritized white patients over black ones when determining who needed extra medical help.
While AI has been cited as a data-driven technology that doesn’t make decisions based on emotions, but on actual facts – the reality is that the facts can be misleading.
In the above example, race wasn’t a deliberate factor in how the AI algorithm reached its decision. It actually appears to have used predictive analytics based on patients’ medical spending to forecast how sick patients are.
Yet, the problem is that black patients have historically incurred lower healthcare costs than white patients with the same conditions, so the algorithm put white patients in the same category (or higher) than black patients whose health conditions required much more care
Bias is inherent in a lot of things we do and often, we just don’t realize it. In this case, the data assumed that people who paid more for services were the sickest. As illustrated, we have to be considerate of the data we use to train algorithms, Cost of services or amount paid shouldn’t be information we use to determine who is sicker than another.
In another example, if skin-cancer-detection algorithms are typically trained on images of light-skinned patients, they would be less accurate when used on dark-skinned patients, and could miss important signs of skin cancer. The data must be inclusive to provide the best results.
While AI can accelerate disease diagnoses, bring care to critical patient populations, predict hospital readmissions, and accurately detect cancer in medical images, the example illustrates the caveat: AI bias –whether because of a lack of diverse data, or the wrong type of data – exists in healthcare and it can lead to social injustice, as well as harm to patients.
In addition to racial bias, unchecked algorithms can cause other types of bias as well, based on gender, language or genealogy. In fact, according to IBM research, there are more than 180 human biases in today’s AI systems, which can affect how business leaders make their decisions.
As an example of gender bias in healthcare, for many years cardio-vascular disease was considered a man’s disease, so information was available based on data collected from men only.
This could be fed into a chatbot and lead a woman to believe that pain in her left arm was less urgent – possibly a sign of depression – with no need to see a doctor right away. The consequences of this oversight could be devastating.