Aug 10
2020
Diagnosing Bias In Healthcare AI: Five Best Practices
By Carlos Meléndez, COO, Wovenware.
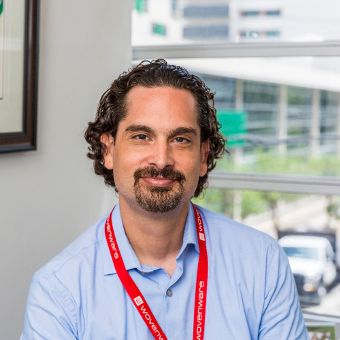
A recent Wall Street Journal article pointed to a biased algorithm widely used in hospitals that unfairly prioritized white patients over black ones when determining who needed extra medical help.
While AI has been cited as a data-driven technology that doesn’t make decisions based on emotions, but on actual facts – the reality is that the facts can be misleading.
In the above example, race wasn’t a deliberate factor in how the AI algorithm reached its decision. It actually appears to have used predictive analytics based on patients’ medical spending to forecast how sick patients are.
Yet, the problem is that black patients have historically incurred lower healthcare costs than white patients with the same conditions, so the algorithm put white patients in the same category (or higher) than black patients whose health conditions required much more care
Bias is inherent in a lot of things we do and often, we just don’t realize it. In this case, the data assumed that people who paid more for services were the sickest. As illustrated, we have to be considerate of the data we use to train algorithms, Cost of services or amount paid shouldn’t be information we use to determine who is sicker than another.
In another example, if skin-cancer-detection algorithms are typically trained on images of light-skinned patients, they would be less accurate when used on dark-skinned patients, and could miss important signs of skin cancer. The data must be inclusive to provide the best results.
While AI can accelerate disease diagnoses, bring care to critical patient populations, predict hospital readmissions, and accurately detect cancer in medical images, the example illustrates the caveat: AI bias –whether because of a lack of diverse data, or the wrong type of data – exists in healthcare and it can lead to social injustice, as well as harm to patients.
In addition to racial bias, unchecked algorithms can cause other types of bias as well, based on gender, language or genealogy. In fact, according to IBM research, there are more than 180 human biases in today’s AI systems, which can affect how business leaders make their decisions.
As an example of gender bias in healthcare, for many years cardio-vascular disease was considered a man’s disease, so information was available based on data collected from men only.
This could be fed into a chatbot and lead a woman to believe that pain in her left arm was less urgent – possibly a sign of depression – with no need to see a doctor right away. The consequences of this oversight could be devastating.
Keeping Human Judgement In the Loop
AI is clearly playing a critical role in healthcare. According to Accenture, hospitals will spend $6.6 billion on AI by 2021. Yet in order to make sure biased algorithms don’t get the final word, human intelligence must always remain involved, using AI to support human decision-making, not overtake it.
The current pandemic shines light on the supporting role of AI, along with its limitations. While it has served a role sorting through huge data-sets in search of a vaccine or treatment, as well as identifying patterns in data to predict future outcomes, we’ll never rely on AI to eradicate the virus. It can, however, augment bright minds and take away some of the grunt work.
The same human logic, or gut instinct should be applied to every decision made by an algorithm as a means to monitor unintentional bias. Past experiences, hunches and situational awareness need to be included with the data mix.
Unbiased Algorithms Begin with Diversity in AI Development
The AI ecosystem is no different than the real world – diversity is the key to well-functioning algorithms and requires diverse data-sets, as well as diverse groups of data scientists to create fair solutions.
Consider the following five best practices for achieving unbiased healthcare algorithms from the outset:
- Start with a diverse workforce. When looking to fill key data science and other decision-making positions, consider a candidate’s broad-based skill set, as well as his/her well-roundedness and ability to empathize. A good candidate for a tech position can no longer rest on his/her tech capabilities alone.
- Groom the next generation. Because of a data scientist shortage there’s more need than ever for education and training of the next generation of professionals. Invest in programs that promote data science training, offering grants and scholarships to encourage greater interest in the field among diverse communities.
- Offer diversity training. While internal (and external) data scientists may have all of the required technology certifications, ongoing sensitivity and diversity training, as well as forums where all staff can speak openly and share their viewpoints and life experiences will help them become more open-minded and considerate when training data-sets.
- Test for bias. Make sure to incorporate bias testing into the quality assurance processes of AI algorithms. Currently, bias identification tools can help to make algorithms more accurate, but they don’t necessarily tackle the root causes of bias, which are larger systemic issues and inequities.
- Make periodic check-ins. Although the majority of the biases arise while training an AI model, unintentional biases can appear over time. Data scientists should regularly check-in with the AI solution and add new data-sets to eliminate growing bias at the source.
Regulation is Required
Just as the U.S. FDA requires drug or medical device manufacturers to conduct clinical trials and undergo federal oversight before their products are commercialized, so too should the government intervene in making sure that AI tools are safe and effective.
There must be standard, ethics-based governing principles to protect patients, ensure that AI tools are designed and developed using transparent protocols, and that patients understand the role AI played in their diagnosis or treatment, as well as the risks and benefits of AI technologies to make informed medical decisions.
The good news is that initiatives from both public and private sectors are underway. Legislators in Washington, DC, are taking a closer look at racial bias in health care algorithms, and last year, NeurIPS ran a workshop to address fairness in AI within health applications. In addition, the Alliance for Artificial Intelligence in Healthcare was formed to advance the safe use of AI in medicine.
Given the growing emphasis on racial and gender equality, the AI technology that is being used to support the work of medical practitioners must be created to enable fair and unbiased medical decisions. This requires a diversity mind-set from data scientists, curious healthcare professionals who trust their gut instinct and regulatory oversight to keep everyone in check.