Nov 8
2022
Closing the Education Gap: Integrating AI Into Your Practice
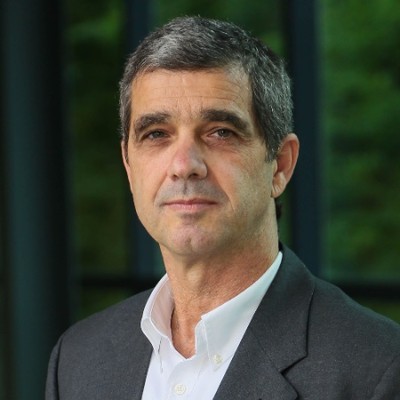
By Ronny Shalev, PhD, CEO and co-founder, Dyad Medical, Inc.
Artificial intelligence (AI) is a driving force in the future of technology, often enhancing the speed, precision, accuracy and effectiveness of human efforts. As a result, AI has had a tremendous impact on nearly every working industry, including healthcare and its specialties. In recent years, there has been an increased adoption rate of AI in the healthcare industry.
Although this growth may be related to the need for telehealth and other remote tools as a result of the COVID-19 pandemic, recent estimates predict a more than tenfold growth in the market for AI in medical imaging over the next decade.
Although there is a projected growth for AI in healthcare, if this technology cannot be efficiently implemented into existing daily workflows, then these AI tools will not be practical in a real-life setting. Understanding how healthcare practitioners use AI in a clinical setting and how AI can help solve real-life challenges are crucial to increasing further adoption rates. This will ultimately drive innovation around this technology and will lead to improved quality of patient care.
What clinicians want from AI-powered technology
A recent survey by the American College of Radiology (ACR) indicated that 30% of respondents use AI to enhance image interpretations across all modalities. The modalities most commonly identified were computed tomography (CT) scans and mammography scans. When asked what they would specifically like AI technologies to do to enhance their clinical practices, respondents indicated that lesion detection (73%) and anatomic measurements (71%) were most important.
These responses indicate that clinicians are most interested in reducing the need for manual tracing and measurements of medical imaging, which takes a significant amount of time and effort. The survey’s findings also indicate that respondents would prefer additional support, or a “second opinion”, when detecting and identifying lesions – a task known to be difficult. The clinicians’ responses also indicated that the development of a method to evaluate an AI algorithm within the workplace setting before purchase is of utmost importance.
With these findings in mind, software developers need to prioritize building technology to help provide a practical solution to clinicians’ most important needs. The use of artificial intelligence may be the best way to ensure clinicians’ needs are met in an effective and practical manner. With the use of AI, the software can continuously learn from collective insights of multiple experts, effectively offering practitioners thousands of “second opinions.”
This type of technology can also help to provide interpretations and analyses of medical imaging, significantly reducing the amount of time it takes for clinicians to measure and trace these images. With less time and effort spent on interpretation and analysis, clinicians are able to spend more time with patient facing tasks – ultimately leading to higher quality care for a lower cost while reducing employee burnout.
Although these solutions exist, there are still several barriers that prevent the successful implementation of AI in clinical practices from occurring. For example, clinicians want to ensure that the AI is safe, effective and solves specific needs before the technology is purchased. However, of those providers who currently use AI in their practice, most were satisfied with their overall experience and found that AI provided value to them and their patients. Therefore, it seems that education about the potential benefits of AI in all practice types will continue to be important (Allen et al, 2021).
Educating others about the benefits of AI
In 2018, the National Institutes of Health hosted a workshop promoting the advancement of AI from research to clinical practice. Four specific areas were discussed to facilitate the use of AI in clinical workflows. First, creating structured AI use cases that define specific clinical needs. Second, making clinical image data available for AI development. Third, ensuring AI safety and efficacy before and after deployment in a clinical practice. Finally, developing standards for clinical integration of AI into routine practice.
It is clear that AI developers must address these four concerns when discussing the implementation of their technology into existing clinician workflows. Healthcare practitioners don’t see themselves as specialists in types of technology, rather they view themselves as specialists in their field of medicine. Therefore, developers who are working to create medical technology should focus on the user – medical practitioners – and how the technology fits in their workflows. One approach for imaging technology to achieve this is to primarily focus on the organ rather than the imaging modality. This would enable the platform to have a very well-defined set of use cases. With this in mind, it should be a developer’s mission to collect many diverse images from multiple sources, which would make its AI computation engine generalizable and robust.
Medical technology developers should also take a priority in receiving annotations from multiple experts around the world to minimize any potential human bias. Doing so puts an emphasis on safety and efficacy, which helps supplement a practitioner’s abilities and decision making.
To address the issue of standard development for clinical integration, the company establishes a process with its collaborators so that full efficiency and benefits are derived from the system without interfering with the normal flow of work in the organization. This process must take place and be tested in various scenarios to make sure its usefulness and efficiency.
Finally, medical technology software should show a return on investment in multiple settings, an area that companies are most interested in, especially in small practices. Furthermore, the technology should provide a way for organizations to evaluate its AI algorithms within the specific organization, even before integrating the platform into its system. As a result, the evaluation process is accelerated even before it involves one of the biggest stakeholders, the user’s IT department.