Jan 23
2017
How the ACA Changed Healthcare Analytics
Guest post by Syed Mehmud, associate of the Society of Actuaries (ASA), MAAA, FCIA, Wakely Consulting Group.
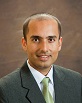
The Affordable Care Act (ACA) produced a wealth of data from its first two years in operation. Health actuaries voraciously consumed that data, using predictive modeling techniques to solve healthcare industry problems that have never been seen before. While we don’t yet know how the ACA may change, I know actuaries will find solutions, because we thrive in the realm of the uncertain.
Actuaries have always been in the business of data. Centuries ago the work involved scanning clerical ledgers to create the first mortality tables. Today, human activity, including healthcare, is far more complex. Every two days, we create more data than was created from the dawn of civilization through the year 2000[1].
A significant portion of my recent work has involved studying ACA data, particularly deconstructing a health plan’s performance using the prism of risk adjustment.
Risk adjustment used to be a niche on the spectrum of a healthcare actuary’s work. However, since the ACA risk adjustment program is now a permanent fixture – for the time being – in commercial individual and small group markets, it is the focus of many actuaries’ every day work. Risk adjustment involves adjusting a health plan’s revenue based on a measure of morbidity of the average member enrolling with the plan. It aims to mitigate incentives to select low-risk populations, and instead re-focus the basis of competition on other factors such as quality, efficiency, and benefits delivered.
The program presents a great opportunity for actuaries to apply predictive modeling concepts on large scale data to deliver actionable insights to clients and employers. From the predictive modeling work, actuaries have learned that risk adjustment renders seemingly intuitive notions of health plan performance and profitability rather meaningless. For example, sicker and costlier individuals may have threatened a health plan’s viability in the past. But that may not necessarily be the case going forward.
At Wakely Consulting Group, my team developed a new data analytics model to parse and understand the drivers of financial performance in the ACA market. Our model revealed that sicker/costlier members are typically overcompensated for the risk that is assumed. It also found that richer metallic tier plans (e.g., platinum, gold) struggle to perform, and variables such as network size and income play an important role in relative profitability. By deconstructing the impact of isolated variables, and reconstituting this impact in the overall financial statement, an organization can measure and manage its risk.
In addition to a top-down view of financials, we learned that we need to understand how a health plan’s experience is evolving at a member-level to provide valuable insight to insurers navigating the financial complexities of the ACA. In this manner, actuaries can help identify segments of the business that are underperforming, or craft a strategy to survive and even thrive in rocky markets.
There are many models of risk adjustment and they change over time. We are almost done analyzing 2015 data to see what lessons can be learned from that wealth of ACA data and whether unhealthy patients continue to make money for insurance issuers.
I am often asked whether investing in predictive modeling and analytics is the way to “win” today. I look at it differently. You may not necessarily “win” by being up to par on advanced analytics. But you will definitely lose if you are not. Organizations that bridge the gap between data generation and data analysis will outperform their competitors. Health actuaries have a tremendous opportunity to play a pivotal role in this transformation.